USER STORY
GIS helps support the sustainability of riparian ecosystem in the Okavango Delta
Using Esri technology to help conserve the Delta’s biodiversity and ecology
The Challenge
Monitoring is necessary to help conserve the Delta’s riparian biodiversity and ecology which is threatened by deforestation, herbivory, and invasive species.
The Solution
ORI GIS laboratory mapped and predicted potential riparian woodland species using machine learning tools in ArcGIS Pro, particularly the forest based classification algorithm.
ArcGIS Pro’s Forest-based Classification and Regression (FbCR) geoprocessing tool was used to identify and predict other riparian vegetation communities based on the variables used for the prediction.
Who is University of Botswana (UB)?
The University of Botswana (UB) was established in 1982 as the first institution of higher learning in Botswana. UB has three campuses, which include the main campus based in Gaborone, Francistown, and Maun campus where the Okavango Research Institute (ORI) is located. ORI conducts scientific studies that inform decision-making on the management of the Okavango Delta environment and its surrounding ecological systems. The Delta is a RAMSAR and World Heritage Site and one of the world’s largest and most intact inland wetland ecosystems. In addition to the environmental and biology laboratories at ORI, the Institute’s GIS laboratory supports multidisciplinary research activities with geospatial data acquisition, analysis, training, and intelligent ArcGIS solutions.
The Challenge
Globally, the sustainability of riparian species is threatened by logging and deforestation warranted by infrastructural and agricultural development, respectively. Despite efforts to conserve the Delta’s biodiversity and ecology, many threats such as deforestation, herbivory, and invasive species persist. Riparian vegetation plays a significant role due to their provision of ecosystem services and products to both human and animal populations. Many ecosystem services provided by riparian tree species are based on their primary production, which includes provision of food, nutrients and water purification.
The Solution
The need to conserve these ecosystems is necessary. To do so, we first established their status in terms of composition, species distribution, and structural analysis. ORI GIS laboratory mapped and predicted potential riparian woodland species using machine learning tools in ArcGIS Pro, particularly the forest based classification algorithm.
The study was conducted in Seronga area in the Okavango Delta. The Okavango Delta is fed by local rainfall and seasonal floods from the Angolan highlands (Gumbricht et al., 2004). Local rainfall in the Delta is out of phase with the seasonal floods (Bjorkvald and Boring, 2002). Mean maximum rainfall in the area varies between 300 and 550mm/year (Wilson and Dincer, 1976; S.M.E.C., 1976; Wolski et al., 2005). The selection of the study site was based on a number of reasons. First, riparian vegetation in the Okavango Delta as a whole is currently threatened by climate change (Murray-Hudson, et al 2011), high risk of over exploitation of woodland resources such as deforestation, etc.
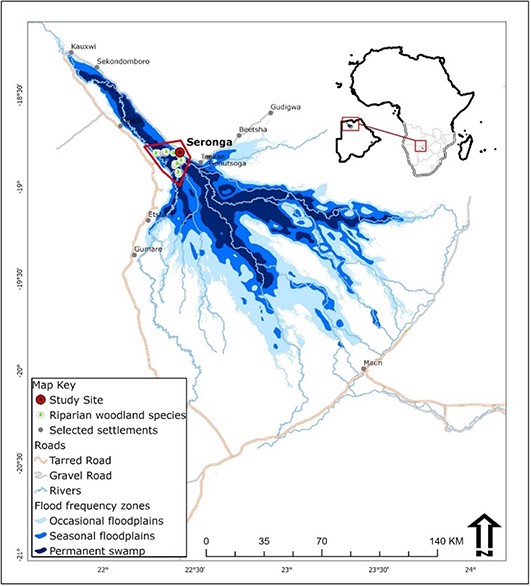
ArcGIS Pro’s Forest-based Classification and Regression (FbCR) geoprocessing tool was used to identify and predict other riparian vegetation communities based on the variables used for the prediction. Variables included a 30m resolution digital elevation raster acquired through the USGS website. Spectral vegetation indices included in the FbCR tool were the Normalized Difference Vegetation Index (NDVI), The Transformed Soil Adjusted Vegetation Index (TSAVI) and Soil Adjusted Vegetation Index (SAVI), all of which were derived from using the Geoye 1 image. In preparation for running the tool, identification of plant communities based on the species composition was performed before any predictions were made. Two dominant plant communities (B and C) were found and used for the FbCR tool (see Fig. 1). The model also included distance to rivers as a continuous variable.
FbCR tool is a new tool only available in ArcGIS Pro in the Spatial Statistics toolbox. This new tool can create models and generates predictions using an adaptation of the random forest algorithm of Leo Breiman and Adele Cutler. Predictions can be performed for both categorical variables (classification) and continuous variables (regression). In our study we predicted riparian communities, so we followed the classification approach. Explanatory variables can take the form of fields in the attribute table of the training features, raster datasets, and distance features used to calculate proximity values for use as additional variables.
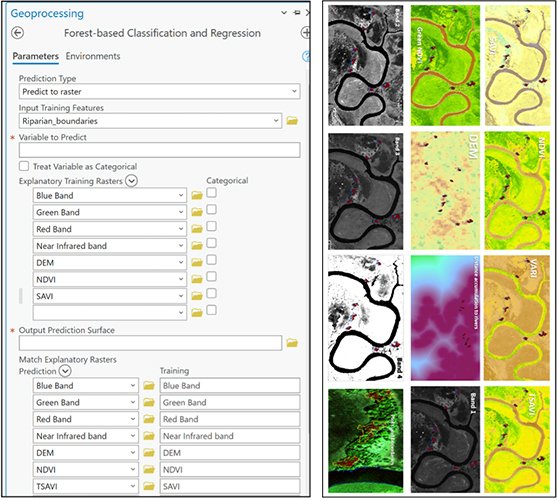
Conclusion
Among others, riparian vegetation in the entire Okavango Delta is currently threatened by climate change (Murray-Hudson, et al 2011), high risk of over exploitation of woodland resources such as deforestation induced by the demand for firewood and planks for the construction of houses and traditional canoes (dugout canoe)
FbCR tool successfully predicted new areas (in a form of raster’s) riparian vegetation in the area. However, individual plant communities were not predicted. Based on this finding, the same methodology can now be applied for the entire Okavango Delta to predict new areas of riparian vegetation.
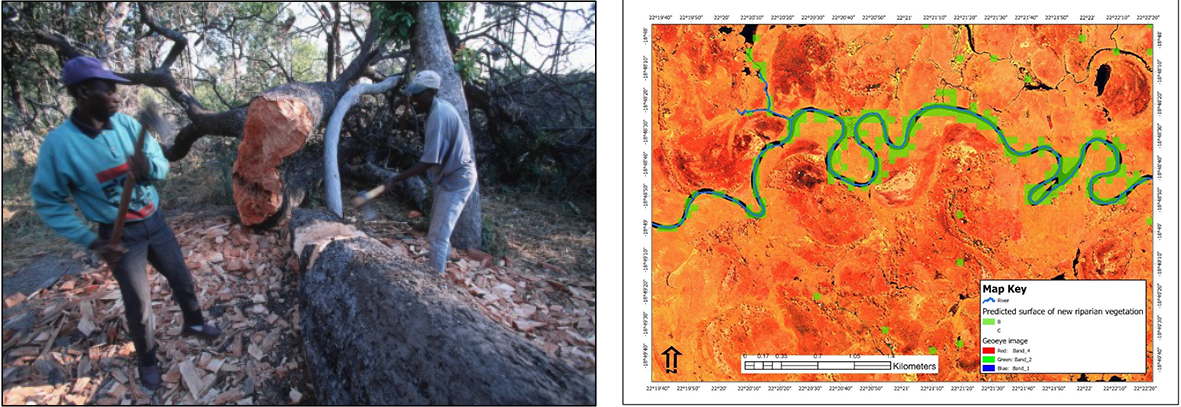
For more information please contact Anastacia Makati at [email protected].
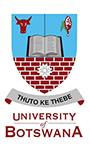